Doctor versus machine: how AI will inevitably change medical diagnostics
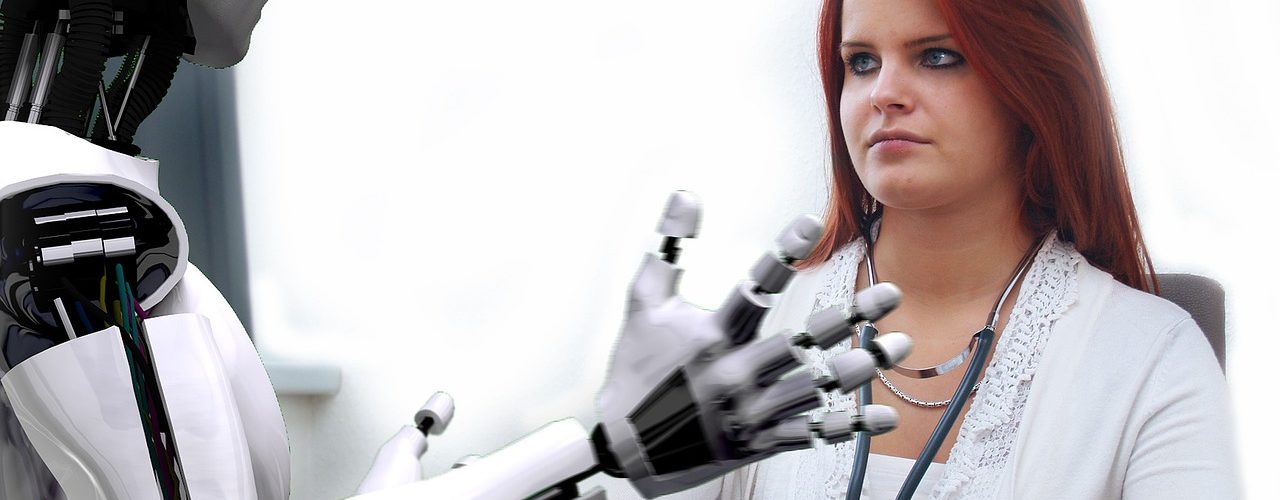
A machine is medically diagnosing a human – it sounds straight out of a science fiction movie, but for everyone that has watched at least one Star Trek movie, the idea is far from new. With artificial intelligence (AI) on the rise, this fiction is becoming reality. In new studies, deep learning, a subset of AI, has already been successfully used to diagnose illnesses like Alzheimer’s disease1 or lung cancer2.
So, what is artificial intelligence? And what is this deep learning everyone is talking about?
Artificial intelligence is engineered intelligence that mimics human information processing and decision making. AI is already extremely helpful in our daily lives. As an example, calculators are taking over solving arithmetic problems for us. Still, the calculator needs to be programmed by humans to be able to fulfil this task. This requires the knowledge of how calculations work on a fundamental level. If the problem gets more complicated, for example determining if a photo shows a cat or a dog, programming a machine to find the solution gets tricky. For instance, what universal parameters make you able to distinguish between the animal and the background? For us, recognising a dog or a cat is easy, although notable exceptions exist (Instagram: @atchoumthecat). Since birth, the neurons in our brain were trained to find the solution. Our parents pointed in picture books at different animals and maybe even amplified the learning experience with a more-or-less accurate audio demonstration.
The principle of how humans learn is used in deep learning which is a subset of AI. Here, a so-called artificial neural network is built that is trained to process input data to determine a prediction for a specific problem. It consists of artificial neurons that process information with parameters that are informed by the experience of the network. To take the example from above: if we want a neural network to be able to distinguish between a cat and a dog, it needs to be trained. For that, in theory, one would use example photos of cats and dogs and let the neural network make a prediction for each. Then the neural network re-assesses the internal parameters that led to the final decision based on the ground truth that is provided (“this is a dog/cat”). This way, photo by photo, the neural network gets better with its predictions. The accuracy of a neural network is dependent on the complexity of the problem, the experience of the network and, of course, on how the neural network is built, which is a science in itself.
The basic idea behind deep learning is not new, but big data and recent advances in both hardware and software have made it into the “hot topic” it is today. So far, little is known about the impact AI will have on our lives. We just have to watch US senators struggle in interviewing Mark Zuckerberg about Facebook’s practices to question politics’ ability to keep up with advances in AI research. The fear that AI somehow will eventually aspire to enslave humankind to reach global domination was fueled extensively by pop culture. Popular Hollywood movies like The Matrix depict a new world order in the future, in which machines take over, enslaving humans to provide energy by keeping them unconscious in glass tanks filled with slime. The question is what could motivate AI to be harmful to humankind when it (presumably) lacks human emotions like greed or vindictiveness? Researchers can think of two scenarios: AI could be programmed to do harm, or it could be programmed to be beneficial, but the way it is conducting this goal is harmful. Accordingly, Stephen Hawking, Elon Musk & co. urged the research community in an open letter in 2015 to extend interdisciplinary research into how AI can be used with the focus to maximise the societal benefit3.
If it comes to medical diagnostics, the benefit seems quite clear. Artificial intelligence could be used in a variety of medical fields to diagnose illnesses and gradually replace the doctor. It is time-efficient, cost-efficient (if mass produced), and ultimately, AI has the potential to outperform human decision making. Currently, 1 out of 6 patients is misdiagnosed by doctors in the UK, meaning that the patient was either diagnosed wrongly, not at all or too late4. It was estimated that by 2020 the NHS will have to pay £3.2bn per year to cover claims alone5. Due to the NHS crisis and a rise in law firms that offer no-win-no-fee deals, the number of claims is likely to increase further. AI does not feel stress or exhaustion during a long shift. It also works 24/7. It can be trained on the basis of big data like no human could. Even if AI is “only” used as a support tool for clinicians to make the final medical decision, the potential to increase the quality of medical diagnosis is high.
In medicine, imaging is often the tool that is used to identify a variety of conditions. For instance, dentists check our teeth with X-rays, doctors use magnetic resonance imaging scans (MRIs) to exclude brain injuries and ultrasound imaging to study the development of a foetus. There are already examples in medical imaging where deep learning was successfully used to diagnose illnesses. Recently, in cooperation with hospitals in China and the US, the start-up 12 Sigma Technologies, developed an algorithm for the detection of lung cancer. For that, a neural network was trained with 500 CT scan images6. Then, doctors in China used the network after checking CT scans for lung cancer. As of now, the machine is able to make a prognosis 5 times faster than the specialist, often even detecting small nodules in the lung that were missed by the doctor. The aim is to train the machine further using the feedback of doctors.
Deep learning was also used in the diagnosis of Alzheimer’s, an illness that can be detected by using a combination of MRIs and positron emission tomography (PET) scans. Alzheimer’s is often diagnosed at a late stage when the patient is already incapable of navigating through the challenges that come with the illness independently. In a study conducted at the University of California, scientists trained a neural network with 90% of a 2,100 PET brain image databank to identify Alzheimer’s disease7. 10% of the databank was used to verify learning success. Then, the researchers tested the network on 40 images where the diagnosis was unknown. Here, the network was able to detect all Alzheimer’s patients correctly. Moreover, it was capable of detecting the disease, on average 6 years prior to the actual diagnosis, which showed that the network was better than the specialists in spotting subtle patterns in the brain images.
In another example, Google used AI to determine the risk of cardiovascular disease for a patient on the basis of a single retinal image8. Currently, risk factors like the patient’s age, smoking habits, body mass index and blood pressure are used in cardiovascular risk calculators, but these data are often not all available from the patient. By using a retinal image, the machine was able to successfully predict the risk of cardiovascular disease within the next 5 years for 7 out of 10 patients. Astonishingly, in 97% of the cases, the trained network was even able to determine the sex of the patient, something clinicians are unable to do9. Although the researchers looked at the areas in the retinal images that made the machine decide the gender, it is still unclear how the network does it. This shows that deep learning can not only outperform specialists but also has the potential to identify patterns that we yet have to discover ourselves.
Of course, there are ethical implications to using deep learning. If algorithms get more powerful in predicting all kinds of diseases just by, for example, analysing our speech patterns10 or our facial expressions11, we need to take measures to protect the data we share on social media or by using our smartphone. It is not just a matter of avoiding personalised advertisements anymore. With the power of AI, health insurance companies could use our health prognosis to exclude us from their schemes or employers could include it in their employment decision.
In medical diagnostics, it is also of interest who is liable in the case of misdiagnosis if the doctor gets replaced by the machine. It is likely that at one point, AI will diagnose illnesses based on parameters that humans do not fully understand. If a company builds a product, the company does understand the mechanisms behind its product. If, for example, wrong materials were used in the production, and it can be proven that the company should have known better, it will be liable in the case where people are harmed due to the product. However, if a neural network was trained, there is no way, yet, of really knowing how it works. It is still not clear how the producer of a neural network would be responsible in case of damage12.
Although there is a lot of excitement generated over deep learning in medical diagnostics, there are still many questions that need to be answered before we are actually greeted by an impersonal white robot with motoric movement and creepy smile at our next GP appointment. There are still enough limitations of AI (and robotics, for that matter), but there is no question that with advances in AI, medical diagnostics will inevitably change. Whether that, ultimately, involves a complete replacement of a human doctor remains to be seen.
This article was specialist edited by Katrina Wesencraft and copy-edited by Audrey Gillies.
References
- https://www.rsna.org/en/news/2018/November-December/Artificial%20Intelligence%20Predicts%20Alzheimers%20Years%20Before%20Diagnosis
- https://www.forbes.com/sites/nvidia/2018/03/14/better-cancer-diagnosis-with-deep-learning/#8b17a0f2a7f6
- Research Priorities for Robust and Beneficial Artificial Intelligence: An Open Letter – https://futureoflife.org/ai-open-letter
- https://qz.com/989137/when-a-robot-ai-doctor-misdiagnoses-you-whos-to-blame/
- https://www.theguardian.com/commentisfree/2017/sep/07/lawyers-nhs-medical-negligence-cases
- https://www.forbes.com/sites/nvidia/2018/03/14/better-cancer-diagnosis-with-deep-learning/#8b17a0f2a7f6
- https://www.rsna.org/en/news/2018/November-December/Artificial%20Intelligence%20Predicts%20Alzheimers%20Years%20Before%20Diagnosis
- https://medium.com/syncedreview/its-all-in-the-eyes-google-ai-calculates-cardiovascular-risk-from-retinal-images-150d1328d56e
- https://medium.com/health-ai/googles-ai-can-see-through-your-eyes-what-doctors-can-t-c1031c0b3df4
- https://emerj.com/ai-sector-overviews/artificial-intelligence-dementia-diagnosis-genetic-analysis-speech-analysis/
- https://techxplore.com/news/2018-11-spoken-language-d-facial-depression.html
- https://qz.com/989137/when-a-robot-ai-doctor-misdiagnoses-you-whos-to-blame/