“Everybody Lies” by Seth Stephens-Davidowitz – a review
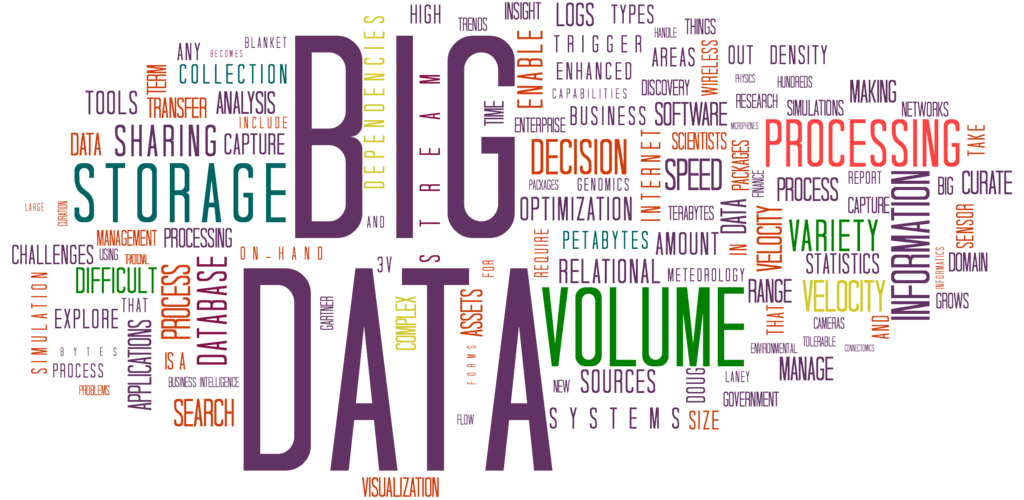
Does the internet know you better than you know yourself? Quite possibly, according to Seth Stephens-Davidowitz, who makes an entertaining case for this in his book: “Everybody Lies: Big Data, New Data, and What the Internet Can Tell Us About Who We Really Are”. It knows what movie you are likely to watch next, your sexual orientation, even whether you’re going to make it to the last chapter.
Stephens-Davidowitz, a former Google data scientist with a PhD in economics from Harvard, argues that big data is providing an unprecedented insight into the human condition. And he backs up his claim with style; bombarding us with case studies, drawing heavily from the holy trinity of internet data: Google, Facebook, and PornHub. The revelations are by turns comforting, unsettling, and hilarious. Some are expected, like the fact that people exaggerate how much sex they are having, some less so, like the unnerving frequency of incest-related Google searches.
First, let’s take a step back. What is big data? Here, it is a loose term used to describe the sheer volume, diversity, and quality of data that modern technology allows us to acquire. Stephens-Davidowitz is concerned with what we can do with this data. He presents what he considers to be the “four powers of big data”, which are revolutionising data science:
the data is honest, novel, abundant, and suitable for randomised controlled trials.
The biggest game-changer is honest data. Stephens-Davidowitz administers his “digital truth serum” in the juiciest portion of the book, which demonstrates that, in contrast to common databases that rely on self-reporting, like polls, new sources of data, such as Google searches, offer an incentive to tell the truth. Google may well be the only reliable source for some information, such as the frequency of self-administered abortions. Stephens-Davidowitz uses Google search data to dispel myths (it turns out social media is less of an “echo chamber” than real life), confirm stereotypes (the working title of this book was “How big is my penis?”) and confront the reader with some uncomfortable truths…
Just before Obama’s re-election in 2012, reports based on polls and surveys indicated that, by and large, race did not influence the voting intention of Americans. However, a study performed by the author revealed a striking correlation between areas with high rates of racist Google searches and areas where Obama underperformed in the election by an amount that could not be explained by other factors. The same pattern was found for Trump’s better than expected performance in the 2016 Republican primaries, with racist searches proving to be the factor most strongly correlated with his popularity. This is a hugely relevant piece of information missing from the debate surrounding race relations in the United States. Google is not only a source of honest data, it is also the only place some confessions see the light of day.
While Stephens-Davidowitz dreams of big data being used to satisfy intellectual curiosity and improve society, he notes that profit-makers have already begun to capitalise on this new resource, exploiting new data in order to manipulate consumers. How do they find the data that is useful (read: honest) to them? Through the relentless application of online A/B tests – experiments in which two random groups of customers are exposed to two different options (A or B), allowing the company to identify the option that produces the most desired response. Why would a social media company ask its users which version of a new feature they prefer – and risk them choosing the option they would use less – when an A/B test would reveal which is the more enticing and therefore profitable? These tests are employed by almost every large online presence you can think of, from Netflix to news outlets; we are all participating in this whether we like it or not.
A/B tests are, in fact, large-scale, online versions of randomised controlled trials carried out by scientific researchers every day. Randomised controlled trials are a type of experiment in which a number of people are randomly assigned to one of two groups. One group is exposed to an “intervention” – this could be a drug, an exercise or anything that is hypothesised to have a measurable effect on the participants – and the other group is not. In medical research, these trials are the gold standard for demonstrating the success of a treatment because they can show a causal link between treatment and patient outcome. With big data comes the power to infer causation from correlation more cheaply and easily than ever before.
New types of data and huge amounts of data – providing sufficient granularity for one to “zoom in” on subsets of the population – complete the four powers. Together, they allow us to answer all kinds of questions, old and new. To demonstrate this, Stephens-Davidowitz recounts an excellent study on the relationship between violent crime and violence in the media, which makes use of copious amounts of box office and crime data. In contrast to the oft-quoted results of lab experiments, which measure increased levels of aggression after exposure to violent films, this study found the opposite effect: a reduction in crime during the weekends when a popular violent movie opened in cinemas. Real-world data is influenced by factors a lab could never account for; from society’s point of view, this study probably has the more ecologically valid result.
Perhaps the most consequential piece of debris left by the big data explosion is the ability to use correlations to predict the behaviour and condition of individuals with increasing accuracy and precision. Pool the Google search histories of people who went on to be diagnosed with pancreatic cancer, and you’ve got yourself a new diagnostics tool. Stephens-Davidowitz believes that “collecting rich data on the world’s problems is the first step towards fixing them”, and the Human Rights Campaign and child protective service agencies are already keen to enlist his help. He has also, of course, been approached about predicting the stock market.
Motivated by the various hazards of this newfound predictive power, the book closes with a warning: with big data comes big responsibility. Correlations are easy to find. However, correlation need not imply causation, and the more you look the more likely you are to stumble upon a coincidental correlation linking two entirely independent variables (as a team who attempted to predict the stock market using Twitter data found out to their dismay1).
There are dangers associated with making decisions based on even the most reliable predictors of behaviour. While most people would support Google’s choice to display the Samaritans helpline to people who search the phrase “suicidal thoughts”, I doubt a policy of arresting predicted criminals before they have broken the law would appeal to many.
Another issue is the rigour, or lack thereof, of big data science. Stephens-Davidowitz appears to take Google search data more or less at face value. He does acknowledge that Google can bring out a different side of people; that searches “skew towards the forbidden”. But is it possible to quantify this effect? I, for one, have expressed many a questionable sentiment in the search box, tempted by an outrageous phrase suggested by the autocomplete, and frequently use Google as an outlet for my morbid curiosity. Nevertheless, the author argues convincingly for the potential uses and likely ubiquity of big data science in the future.
Personally, I find this ubiquity – “everything is data” – to be the biggest concern. Stephens-Davidowitz touches upon it, and concedes that “Big Data does not eliminate the need for all the other ways humans have developed over the millennia to understand the world.” He gives some specific examples of where big data can fail. But what about the long term repercussions?
As more people begin to realise the rewards that can be reaped from big data, there is a danger of reducing almost all aspects of life to a data science. After all, we as a species are primitive data scientists. The instinct to go to the side of the sick in order to care for them helped our species survive for millennia. The idea of isolating some sick people came later, after the observation and analysis of many outbreaks of contagious diseases. In this case we have over-ridden an instinct for our own good. What would society look like if we had more reason to go against our gut? In some areas, such as medicine, this is likely a positive thing. In other areas, it could lead to denying help to individuals who need it because of predicted outcomes, pressure for reduced privacy and less emphasis being placed on in-depth understanding.
What about a society where companies can continually manipulate people’s behaviour unchecked? There is no coherent set of laws to prevent this and many would struggle to put their finger on exactly why they are uncomfortable with it; it’s not explicit control, but a more subtle form, with consequences including social media addiction. I fear we are seeing the effects already.
We have never witnessed the evolution of a species with this power at its disposal. Now, more than ever, we may need to keep a close eye on our own critical thinking and the values we aspire to as a society. At the same time, big data science is providing us with unique opportunities – to expand our knowledge and improve quality of life – outside the domain of traditional science. In addition to offering some tentative hope for the future, “Everybody Lies” is an excellent read if you are interested in data science, behavioral psychology or are just nosy.
This article was specialist edited by Anna Henschel and copy edited by Kirsten Woollcott.
References
- The specific study Stephens-Davidowitz refers to claimed that the volume of Tweets expressing “calmness” on a given day was a strong predictor of the stock values of a number of US companies in the next days. The correlation turned out to be a fluke. Articles from 2010 hailing this “discovery” can still be found online: https://www.theatlantic.com/technology/archive/2010/10/predicting-stock-market-changes-using-twitter/64897/
3 Responses
[…] Motivated by the various hazards of this newfound predictive power, the book closes with a warning: with big data comes big responsibility. Correlations are easy to find. However, correlation need not imply causation, and the more you look the more likely you are to stumble upon a coincidental correlation linking two entirely independent variables (as a team who attempted to predict the stock market using Twitter data found out to their dismay1). […]
[…] Duncan, Anna. “‘Everybody Lies’ by Seth Stephens-Davidowitz – a Review • TheGIST.” TheGIST, 13 Feb. 2019, https://the-gist.org/2018/11/everybody-lies-by-seth-stephens-davidowitz-a-review/. […]
[…] Duncan, Anna. “’Everybody Lies’ by Seth Stephens-Davidowitz – a review.” The Gist: Glasgow Insight into Science & Technology, 9 November 2018, accessed on 25 November 2020, https://the-gist.org/2018/11/everybody-lies-by-seth-stephens-davidowitz-a-review/. […]