Quantum computing and machine learning: a mutually beneficial partnership
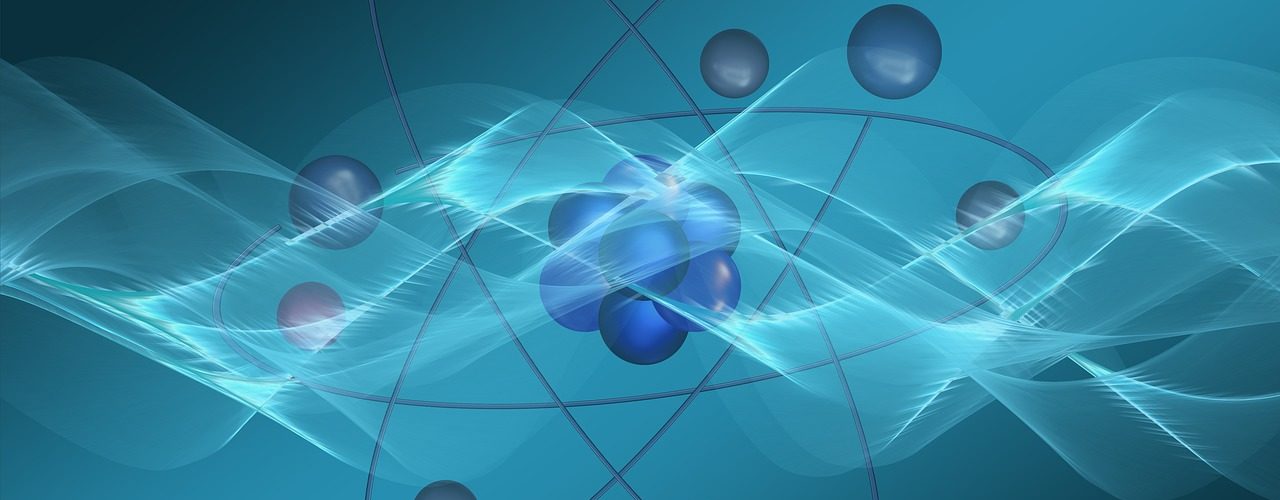
The phrase “artificial intelligence” has been circling in the public domain for several decades and inspires fear and awe. When we think of artificial intelligence (AI), many ideas come to mind, such as people being automated out of jobs, Asimov’s laws of robotics, Terminator, Hal, and other dystopian scenarios pushed in films and other media. Despite this doomsday perception, various forms of AI have now infiltrated everyday life, whether we like it or not. This can bring real benefits (see this previous piece about applications of AI in engineering) but should be used carefully and not seen as a magic solution to all ills. We are now perhaps witnessing a similar story to the development of AI in the recent hype around quantum computers. Previously only viewed as ideas in theoretical physics, quantum computers now exist and run their own algorithms. As with AI, some see this as excellent; others panic. People might imagine scenarios where the knowledge of every programmer becomes obsolete as every computer is replaced with a quantum computer or that a hacker will implement Shor’s algorithm and break the cryptographic methods currently used to keep the internet secure. Again, despite the trepidation from some, the development of quantum computers carries on.
Given the similarities in the perception of the fields of AI and quantum computing, it is interesting to look at how they can work together. The phrase “quantum artificial intelligence” can easily conjure even more dramatic images of the apocalypse than just quantum computing or AI. However, when we look at quantum AI through a more realistic and critical lens, we see that this combination can be very useful. Google even has a team dedicated to researching quantum AI and providing services for current applications of such technology 1. Here we will explore the synergies between the fields of AI and quantum computing and consider how this can be useful.
Before diving into quantum AI, I will make a distinction between “classical” computers and “quantum” computers. For more information about how quantum computers work, see this article from Wired. Classical computers run algorithms using bits, which are values of 0 or 1 represented in the computer’s circuitry, and logic gates, which perform operations on these bits. Quantum computers run algorithms using qubits, defined as a “superposition” of 0 and 1, and quantum gates, which operate on qubits. Creating a physical quantum computer requires manipulating the quantum-mechanical properties of individual particles. If this sounds incredibly complex and difficult to achieve, that is because it is. Here we will not worry too much about the lower level operation of quantum computers but will look at what quantum computers could realistically do with near-term hardware and how AI can fit into the mix.
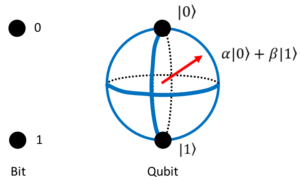
A common graphical representation of a qubit versus a bit, illustrating the superposition of values for a qubit. Image created by author.
“Quantum AI” is a very broad term that can be applied to various methods and applications involving quantum mechanics and AI. Here we will look at three main categories of quantum AI that are currently researched:
1. Classical AI applied to problems in quantum physics
2. Quantum computing enhancements to classical AI algorithms
3. Ground-up design of AI using quantum computing theories
From these categories, it is interesting to note that much of the current quantum AI research uses existing and fundamentally “classical” AI algorithms. These can be applied to problems in quantum systems, as in category 1), or they can incorporate quantum algorithms to improve, for example, the algorithm’s speed, as in category 2). Aside from these more classical AI approaches, category 3) refers to current research in creating entirely new “intelligent” algorithms with quantum computing as their foundation.
First, let us consider how AI can be applied to quantum physics problems. These applications have much the same motivation as other applications of AI: designing and controlling a quantum mechanical system is challenging. This is mainly because any interaction with the external environment affects a system’s quantum-mechanical properties, thereby creating noise in the system and possibly resulting in information loss. We cannot completely detach a quantum computer from the outside world; otherwise, we would not be able to observe any useful information from it. However, we can use AI methods to mitigate these issues, for example by designing more efficient and robust computations for a quantum computer that are less prone to errors, or devising strategies to correct such errors, known generally as quantum error correction.
Now we shall look at how quantum computing can be incorporated into a classical AI algorithm. Quantum computers have specific tasks at which they outperform classical computers in terms of speed; this is commonly referred to as “quantum advantage”. In other tasks, they have no advantage over classical algorithms. One important assumption made when considering quantum enhancements to AI relates to data representation. For the methods shown here to work, it is assumed that data are pre-processed and loaded into a quantum state which can be queried by a quantum computer. Research is ongoing into how feasible this would be in reality. Nevertheless, by considering what quantum computers could do “better” than classical computers, with the assumption on data holding, we can develop a library of “tools” that could benefit classical AI. Some of these are as follows:
– Calculating distances between vectors
– Computing vector inner products
– Solving systems of linear equations
The most common theoretical applications of these tools are for classification and clustering problems. In classification, a computer learns patterns from vectors of features (e.g. measurements of physical quantities or pixel colour values in an image) that belong to certain classes. Using these patterns, the computer identifies the class of new vectors. Similarly, clustering deals with vectors of features that are all unlabelled, and the task is to find groups of features. Both methods require computing distances between all or a subset of the vectors. As the dimensionality of the vector space increases, this becomes much more computationally expensive. It is, therefore, useful to have more efficient methods for calculating distances using quantum computing. Another common method involving vectors of features is Principal Component Analysis (PCA). For its application in AI, the main goal of PCA is to project these vectors in a way which maximises distances between different groups. The process of computing principal components also involves some linear algebra which presents another opportunity for potential quantum advantage.
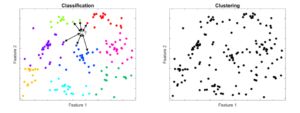
Supervised learning looks at data that are labelled (indicated here by their colour) and works out the label for a new point. Clustering looks at unlabelled data and aims to divide them into groups. Image created by author.
Thus far, we have considered AI and quantum computing separately by applying one to the other. This can be useful as discussed but perhaps does not fully exploit the potential of using quantum computing for creating “intelligent” machines. What if we tried to create new quantum algorithms which can be considered “intelligent”? Certain paradigms in AI which relate more broadly to theories of learning are now being generalised from classical computing concepts to quantum computing. These are presented as sorts of building blocks that could be used to create fully quantum AI. As per the original aims of AI research in the early 20th century, some current research also seeks to replicate human intelligence using computers. This leads to the following question: can we capture some of the key biological processes of the human brain in a computer program? When only using classical computing, it seems the answer is no. Given the underlying processes in the brain are governed by quantum physics, quantum computers could be better suited to this task. Much of the research in this domain is rather metaphysical and philosophical, and some of it is outright pseudoscience. However, simulating aspects of the brain with quantum computers remains a possibility.
So to summarise – will quantum AI kickstart the robot apocalypse and steal your bank details? Not in the very near future, since it is currently difficult enough to make quantum computers do anything, and threats posed by would-be quantum hackers are already well known with measures being put in place to stop them. However, steady progress is being made in quantum computing with the support of AI, which will also help accelerate certain AI methods. Both fields of quantum computing and AI are still developing, and I expect they will continue to be combined and produce more useful and interesting results. Hopefully, full quantum intelligence does not arrive too soon, though, lest my own measly classical AI research becomes irrelevant.