The Poet Inside All of Us
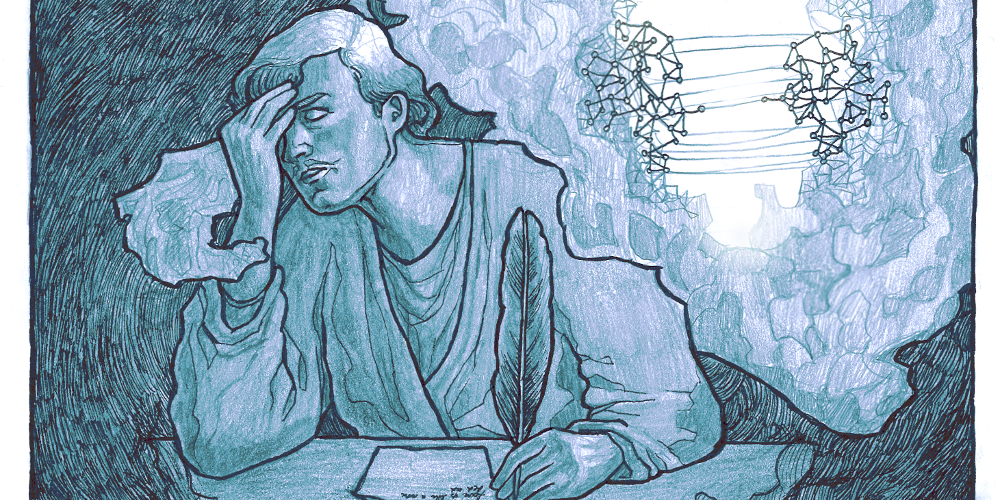
Good metaphors won’t come easily. Most of the time, we fumble awkwardly around our mental lexicon for an apt description, but when all of a sudden it floats up into consciousness, the bliss it brings burns slowly. No wonder then, that ever since the 1960s, the field of Artificial Intelligence has been hard at work to construct analogy-drawing software to which we could offload our struggles with the indescribable. Along with the recognition that good metaphors spring from a core mechanism of abstract thought (one far from exclusive to lovelorn lyricists and crafty rhetoricians) psychologists have increasingly come to rely on such computational models for suggestions of what underpins this fumbling flair for figuration.
Metaphors are pervasive. Every 10th to 25th word or so, we wax metaphorical, often blind to the figurative nature of our own speech. Consider, for example, how our words for “knowing” tend to borrow from visual perception (“insight”, “illuminate”), and how “cogitation” derives from the Latin word for “agitated motion”, as if the thinking intellect was a physical space of objects jostling around 1. Studying the etymology of words, we find ourselves invited to an archeological adventure down a regress of fossilised poetry that traces back to our most concrete sensory experiences. Even “metaphor” itself is a hand-me-down from the Greek phrase for “carrying across”. Trying to think abstractly without this scaffold of 100,000 years’ worth of once-upon-a-time neologisms gives us a hint as to how feeble and impoverished a metaphor-less mind would feel like 2.
This has stimulated a theory, according to which the literary metaphor is a by-product of how abstract concepts come about in the brain by opportunistically re-purposing sensorimotor networks. Brain imaging studies have shown that when subjects read about metaphorical actions, such as “biting the bullet”, the corresponding action-controlling areas in the brain increase their activity, as if its concrete meaning was simulated without actually being acted upon. The brain lights up as if it were actually biting a bullet! 3. Moreover, “dead” metaphors – those we no longer consciously recognise as figurative – engage the motor system less than novel ones, as if, with time, metaphors cease to piggyback on pre-existing circuitry, and eventually become their own schemas 4. But why are some networks chosen and not others?
Our intuition of metaphor as a transfer is reflected in models that state that metaphor occurs when two mental structures, “analogues”, are mapped to each other, so that corresponding parts become aligned. If one of these analogues lacks components found in the other, a brain algorithm ensures that it borrows this neural structure from the richer one. As a result of this “transfer”, we reason metaphorically, and understand one concept in terms of the other. In the above example, when the expression was coined, “biting the bullet” was a relatively rich concept, since soldiers in the past bit on bullets to endure painful surgery without anaesthetics. At some point, Victorian gentlemen appropriated this sensorimotor network to flesh out their concept of courageously accepting life’s hardships 5 .
Another example is the metaphor “Life is a Journey”. In a simulation called the Structure-Mapping Engine (SME), “Life” and “Journey” would be inputs. The latter would be encoded to contain logical statements such as “peaks are higher up than valleys”, to represent how a terrain has ups and downs. “Life” would have a parallel statement for happiness and depression, with “higher up than” corresponding to “better than”. An algorithm searches for such local matches, for example mapping “Traveler” to “Living person”, and “Destination” to “Death”. Then, if “Life” is missing any structure that’s in “Journey”, like “valleys are followed by peaks”, this structure is transferred to it. It’s quite like how we, by comparing life to a hill-climbing odyssey, can come to the uplifting conclusion that for every valley there’s a peak and, upon reaching an all time low, decide to keep on walking 6 .
The idea that “the mind is a computer” is itself a metaphor; an enormously profitable one, but, like any paradigm, is bound to fall out of favour. Long-term memory isn’t literally an orderly filing cabinet, filled with strings of symbols that a processor traverses for a match. Instead, the now-dominant view is that concepts in the brain are overlapping, distributed activity patterns. In order to build a model that represents more closely the fatty, neuronal forest inside your skull-case, many computational modellers instead use networks of neuron-like units. The connection strengths of these units are adjusted based on how well their initially random predictions fare. By constraining future activation spread this way, after a great number of iterations, the network can be “trained” to recognise patterns 7.
An example of such a network-based architecture closer to biological reality is LISA (Learning and Inference with Schemas and Analogies). LISA, unlike SME, represents logical statements as activity patterns, by letting concept-units fire in synchrony. For example, the concept “Shakespeare” could be represented by the units human, male, dead… and the role “playwright” by job, words, and so on. The statement “Shakespeare is a playwright” is formed by their synchronous activity. As a result, when the “Shakespeare” network is activated, it will unleash a cascade of firing patterns among the constituent concept-nodes, which in turn excite other networks they are part of, such as the statement “Chekhov”+”playwright”. Based on this co-activation, an algorithm will then strengthen this mapping, in effect adding it to LISA’s stock of analogies. While this certainly makes LISA no Shakespeare, experiments have shown that its performance corresponds well with that of human subjects 8.
Then again, the brain isn’t really a stationary logic-machine, humming singly and silently inside its silicon cocoon, but a body organ embedded in an environment that it actively intervenes in. For networks, like those in LISA, to fire in synchrony, then usually the environment must trigger them at the same time. For example, while studying a child’s utterances, researchers found that the metaphor “Knowing is Seeing” is acquired after a period of conflation, in which the child is unable to differentiate between the two, because they co-occur in phrases like “Let’s see what’s in the box”. “Warmth” similarly acts as a source analogue for “Affection”, because a mother’s embrace also provides warmth, and “Time”, based on correlations between distance and duration, is conflated with movement in space, either as an object moving towards us (“An upcoming deadline”) or as something that the self moves relative to (“Approach the deadline”) 9 .
These theories, which emphasise bodily experiences, have spawned a new type of models, which simulate intervention in the environment. For example, one network was trained to spatial relationships such as “If blue is to the left of red, then red is to the right of blue”, and the effect of its own movement in space by asking it what color it would see from its current position. The network was subsequently trained on time relationships, but less richly so. Nevertheless, it succeeded to make inferences about time by relying on its knowledge of space – just like we do when we talk about “upcoming deadlines”! 10.
While an analogist bot is unlikely to win the Nobel Prize for Literature anytime soon, perhaps it would have a shot at the Turing Prize. Many philosophers attribute the bottleneck within Artificial Intelligence to a failure to appreciate how environmental interactions determine our metaphorical constructions, and consequently also our thoughts. Maybe to gain the full standard set of embodied metaphors, there simply is no shortcut other than to live a human life – to travel that journey. Regardless, the theory of conceptual metaphor has a delightfully unifying quality to it, and immense intuitive appeal, and by using computational modelling, makes a strong case that there is a poet inside all of us. Not literally of course, but you know what I mean.
This article was specialist edited by Yulia Revina and copy edited by Nicole Nayar.
References
- More on http://www.etymonline.com/index.php?term=cogitation
- For a really great, easy-to-read book about the role of metaphor, check out “I is An Other” by James Geary (New York: Harper Perennial. 2011)
- Boulenger, V., Hauk, O., & Pulvermüller, F. (2009). Grasping ideas with the motor system: Semantic somatotopy in idiom comprehension. Cerebral Cortex, 19, 1905–1914. This research is discussed in Benjamin K. Bergen’s “Louder than words” (New York: Basic Books. 2012.)
- Desai, R. H., Binder, J. R., Conant, L. L., Mano, Q. R., & Seidenberg, M. S. (2012). The neural career of sensorimotor metaphors. Journal of Cognitive Neuroscience, 23(9), 2376–2386.
- For more information about the gory history behind the “bite the bullet” expression, read http://www.phrases.org.uk/meanings/bite-the-bullet.html
- Structure-mapping theory is described in Chapter 6 of “The Analogical Mind: Perspectives from Cognitive Science”, edited by Dedre Gentner, Keith J. Holyoak, and Boicho, K. Kokinov (Cambridge: Bradford Books. 2001).
- For a beautifully written introduction to the different perspectives in cognitive science, check out “Mindware: An Introduction to the Philosophy of Cognitive Science” by Andy Clark (Oxford: Oxford University Press. 2013.)
- LISA is described in Chapter 5 of “The Analogical Mind”
- This is discussed in the fascinating book “Philosophy in the flesh: The Embodied Mind and Its Challenge to Western Thought.” by George Lakoff and Mark Johnson (New York: Basic Books. 1999. Chapter 4.)
- Flusberg, S. J., Thibodeau, P. H., Sternberg, D.A., & Glick, J.J. (2010). A connectionist approach to embodied conceptual metaphor. Frontiers in Psychology, 1, 197.