Uncovering the mysteries of protein structure with AI
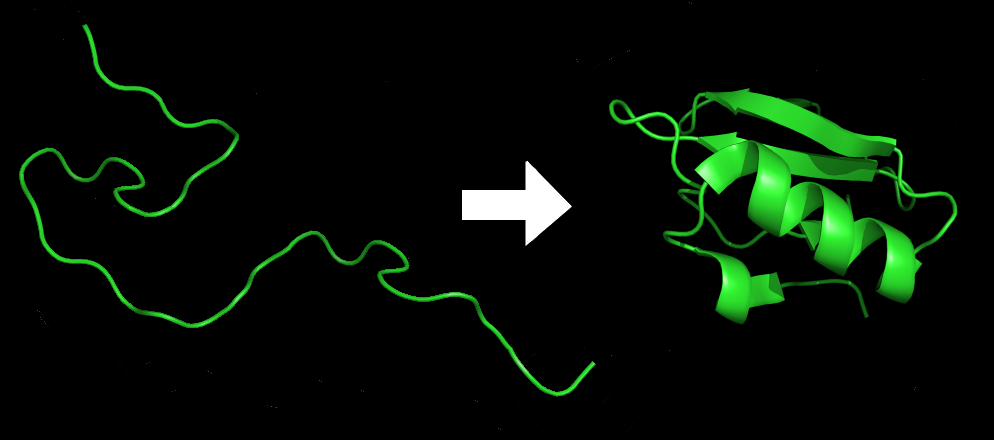
Proteins, essential for all life on Earth, aid in all biological processes. They have a variety of functions including increasing the speed of reactions as enzymes, fighting pathogens as antibodies, carrying messages to control bodily functions as hormones, and many more. All proteins comprise different amino acids joined together to form a chain which is folded into a specific 3D structure that allows it to carry out its function, and sometimes combine with other proteins to form larger complexes[1]. Until recently, the structure of around 83% of these integral biological molecules remained unknown. However, in the past five years, there have been significant developments in solving protein structure and folding using Artificial Intelligence (AI).
Proteins are far too small to see with a light microscope, so scientists have developed several methods to determine protein structure indirectly. The most popular are X-ray crystallography, nuclear magnetic resonance spectroscopy and cryoEM. These involve shooting electromagnetic waves or molecules into the protein, analysing how they exit, and inferring protein structure from the pattern[2]. Solving the structure of a single protein can take months to years of experiments (if it’s even possible) using these methods.
The invention of the computer model AlphaFold in 2018, and more recent extensions, have been revolutionary[3]. This AI model, trained to infer the 3D structure of proteins based on their amino acid sequence, has proven to be highly accurate in inferring structures and has solved 98.5% of human proteins within a year. It takes only minutes and has been a remarkable feat in the field of structural biology.
Understanding protein structure is particularly useful in the production of medicines. Scientists typically produce a chemical compound that will interact and bind with a specific protein in our body, altering its function and resulting in a desirable physiological effect. For example, ibuprofen works by binding to proteins COX1 and COX2, reducing the production of prostaglandin and prostacyclin, which are responsible for inflammatory responses and sensitivity to pain[2]. Knowledge of COX protein structure aided in creating a molecule that can bind to them and inhibit their effects.
Another AI model, WSME (Wako-Saitô-Muñoz-Eaton), predicts how small proteins will fold from their 2D amino acid chain into their 3D structure[4]. This knowledge aids in understanding and developing drugs for diseases caused by improper protein folding, such as Alzheimer’s disease. A notable development to this model, now WSME-L(SS), can predict folding for proteins of any size, as well as account for disulphide bonds in its predictions, a type of interaction many extracellular proteins (such as antibodies) have which determine their 3D structure.
AI models like AlphaFold and WSME significantly ease the process of solving protein structure. Though not yet reliable enough to put structural biologists out of a job, its technology is rapidly developing and will undoubtedly be responsible for groundbreaking advancements in synthetic biology.
[1] Wakim, S., & Grewal, M. (2021, September 4). Proteins. Butte College. Available at: https://bio.libretexts.org/@go/page/17000
[2] Nelson, D. L., & Cox, M. M. (2017). Lehninger principles of biochemistry (7th ed.). W.H. Freeman.
[3] Tunyasuvunakool, K., Adler, J., Wu, Z. et al. Highly accurate protein structure prediction for the human proteome. Nature 596, 590–596 (2021). https://doi.org/10.1038/s41586-021-03828-1
[4] Ooka, K., Arai, M. Accurate prediction of protein folding mechanisms by simple structure-based statistical mechanical models. Nat Commun 14, 6338 (2023). https://doi.org/10.1038/s41467-023-41664-1
Edited by Despoina Allagioti and Hazel Imrie
Copy-edited by Rachel Shannon