The bots and the bees
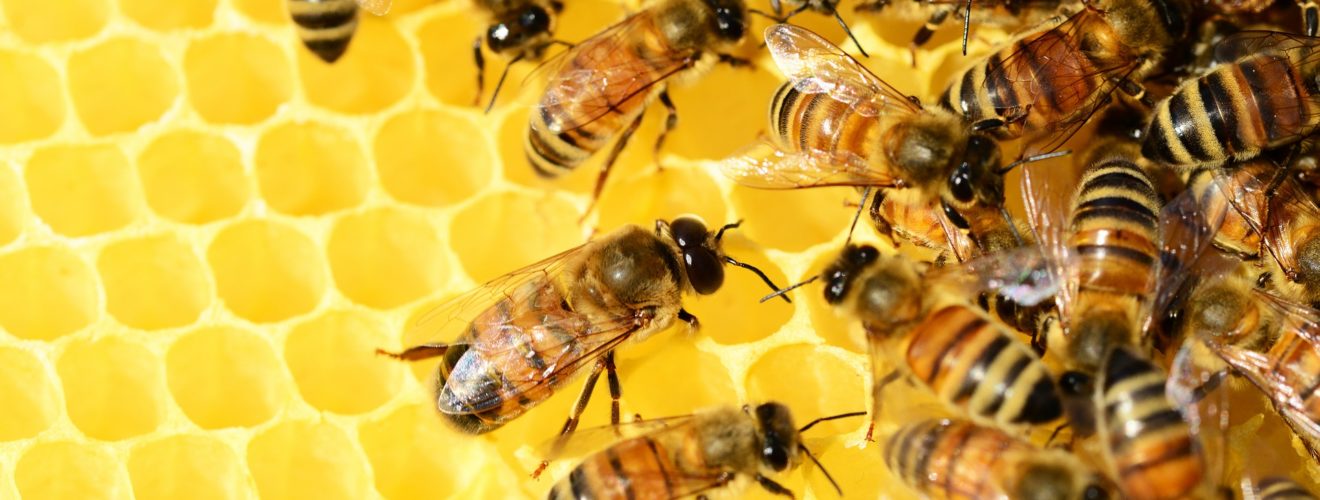
What is intelligence? This is a question humans have been asking since we ourselves became intelligent enough to conceptualise it.
Over the years intelligence has been defined as many different things, including the capacity for learning, self-awareness, planning, creativity, empathy, problem-solving and many other examples aside. However, it has been generalised as the ability to identify information, keep track of that information, and then adapt that information for use within a context. So in the pursuit of creating better and more advanced Artificial
Many therefore argue the next big benchmark for AI is the ability to mimic Animal-level Intelligence as evidenced by the upcoming Animal-AI Olympics1 where AI will be put through a series of tests originally designed for animals in a real-world simulation to see how much progress can be made in the field.
Alongside this, there is research regarding the use of machine learning — the concept of programming an algorithm which allows it to categorise data and make its own connections, rather than designing an AI with preconceived logic — to imprint experiences onto it and allowing it to ‘teach’ itself.
Recently, a Malamute named Kelp was rigged up with a head-mounted go-pro and various motion sensors on its legs and body. Kelp’s movements and vision were then recorded in 380 different contexts2. This experiment was done with the purpose of informing an algorithm with the experiences of a dog, to see if that algorithm could perform 3 separate tasks in the visual world; (1) Acting like a dog (knowing a dog’s limb movements from a visual data feed), (2) Planning like a dog (when shown 2 non-consecutive image frames, predicting a dog’s limb movements in getting from point A to B) and (3) Learning from a dog (when shown a still image, using prior learning from the dog to infer which parts of the image constitute walkable surfaces).
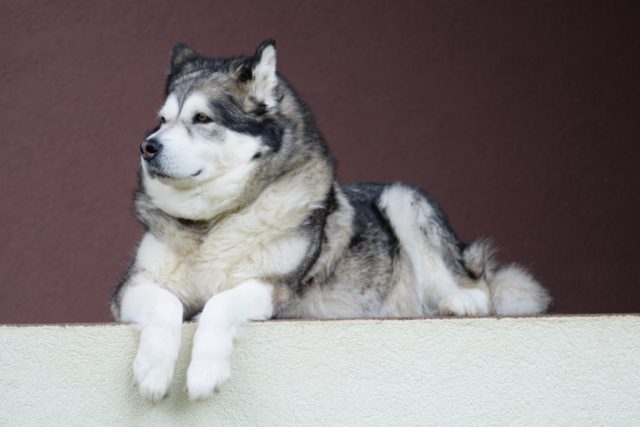
While these first steps towards end-to-end modelling of intelligent agents are incredibly impressive, these visually-trained algorithms don’t yet have the same range of problem-solving abilities as a canine.
This dog analogy
This does lead us to ask, if we can’t presently fully model an animal-like intelligence, in what other ways can an AI interact with our animal friends?
Colony Collapse Disorder (CCD) is a syndrome that is destroying beehives the world over. This is when all of the worker bees within a hive simply… abandon it, leaving the queen, the infrastructure and the food behind to rot. This has cascading effects, with crop failures and entire food chains being destroyed without bees to pollinate the surrounding territories. While no single cause can be blamed, many people have come to believe that Varroa mites are a key indicator that appears before CCD occurs. Unfortunately for beekeepers, these parasites are minute, bees move quickly enough that they’re hard to focus on, and the sheer numbers involved make it difficult and time
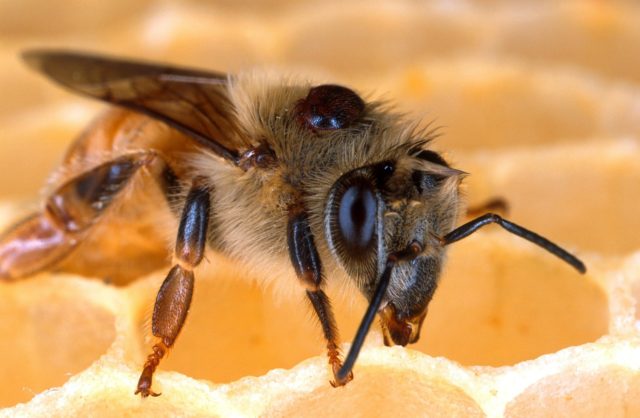
Other bee-related problems are also being tackled by start-ups using AI and machine learning. To prevent swarming, the World Bee Project teamed up with IT Firm Oracle to create a network of smart hives over the world6 that use acoustic data from sensors placed on participating apiaries. With this, they can attempt to construct a global idea of bee colony health by listening to the different sounds that hives produce in different environments and under different pressures, with the goal of using this mass-data to infer the variables that are causing bee-death worldwide. Should this be successful, it will allow beekeepers to ‘listen’ to their bees to predict and pre-empt any tragedies, and the global community at large by sharing data may tackle these issues together in a wider context.
So fearful are we of the bees dying that many institutions are developing artificial bees to pollinate plants should natural pollinators become critically endangered7. In a maliciously ironic twist, certain corporations that are planning to use these robots may even end up utilising them to spray the very pesticides that are killing their organic counterparts!8
AI helping out bees is, however, not a one-way street! The ‘intelligence’ of bees is somewhat of a mystery, with individual drones showing far better problem-solving skills than something with so few neurons would previously have been expected to. Some interesting insights into the long-term memory and mathematical abilities of bees have been made recently – they understand the concept of zero and can do arithmetic! This has some very interesting implications for AI design in the future according to Associate Professor Adrian Dyer, “If maths doesn’t require a massive brain, there might also be new ways for us to incorporate interactions of both long-term rules and working memory into designs to improve rapid AI learning of new problems.”9
For as long as human beings have been innovating with new technologies, we have taken inspiration from the natural world and it seems like this tradition is going to continue far into the upcoming AI boom. Thankfully, with modern advances in sensors and big-data analysis, it is looking like we will finally be able to start giving back to nature, starting with the bees.
This article was
References
- http://animalaiolympics.com/#intro
- https://arxiv.org/pdf/1803.10827.pdf
- https://theconversation.com/how-understanding-animals-can-help-us-make-the-most-of-artificial-intelligence-74742?xid=PS_smithsonian
- https://actu.epfl.ch/news/using-artificial-intelligence-to-save-bees/
- https://zibees.co/
- https://www.oracle.com/uk/corporate/pressrelease/ai-smart-hives-network-helps-conserving-global-honey-bee-2018-10-16.html
- https://www.pw.edu.pl/engpw/Research/Business-Innovations-Technology-BIT-of-WUT/B-Droid-a-robot-that-s-busy-as-a-bee#
- https://www.cbinsights.com/research/walmart-farming-drones-patent/
- https://www.rmit.edu.au/news/all-news/2019/feb/bees-brains-maths